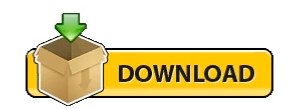
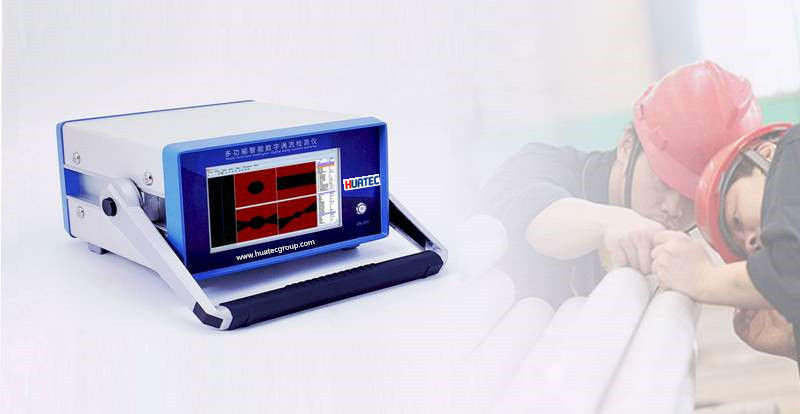
Secondly, ligand-based methods depend on a basic assumption called the molecular similarity principle. Therefore, ligand-based and PCM methods are preferred in most cases. However, the methods have two major limitations: (1) intensive computational complexity and (2) the shortage of 3D structure data for compounds and proteins. AutoDock, Glide, Fred, and AtomNet are examples of docking tools.

Structure-based methods use three-dimensional (3D) simulation for molecular docking to discover CPIs. First, structure-based methods yield reasonable prediction performance and visually interpretable results. In addition to the conventional approaches, proteochemometrics (PCM) methods have been proposed to predict CPIs by incorporating both ligand and target space within a single model. Traditional in silico models can be grouped into two approaches, which are structure-based methods and ligand-based methods. In silico models have emerged to aid traditional experiments by narrowing down the search space and prioritizing molecules with the highest potential. In vitro experiments are commonly used in identifying CPIs, but it is not feasible to discover molecular and proteomic space only through experimental approaches. As for the targets of the compounds, there are about 200,000 reviewed human protein records. Each space is enormous and heterogeneous, moreover, most of the CPIs space remains to be discovered. Exploring both molecular and proteomic space is a highly challenging and cost-intensive procedure.
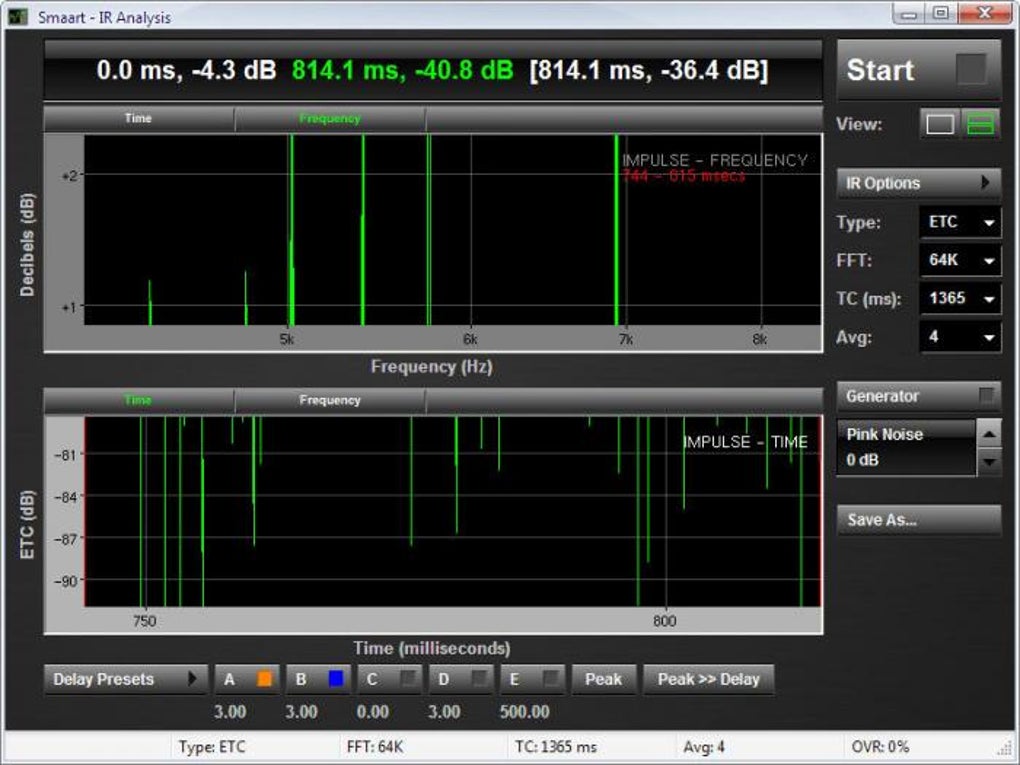
We found that there were significant differences in performance between pretrained models and non-pretrained models.Īnalysis of compound–protein interactions (CPIs) has become an important prerequisite for both discovering novel drugs for known protein targets and repurposing new targets for current drugs. Additionally, we pretrained models on a training task then finetuned them on a test task to figure out whether Multi-channel PINN can capture general representations for compounds and proteins. Therefore, Multi-channel PINN can be advantageous when used with appropriate representations. The experimental results obtained indicate that the multi-channel models using protein features performed better than single-channel models or multi-channel models using compound features. As a proof of concept, Multi-channel PINN was evaluated on fifteen combinations of feature pairs to investigate how they affect the performance in terms of highest performance, initial performance, and convergence speed. To fully utilize sparse public data, we additionally explore the potential of transferring representations from training tasks to test tasks. Multi-channel PINN can be fed with both low and high levels of representations and incorporates each of them by utilizing all approaches within a single model. With representation learning, Multi-channel PINN can utilize three approaches of DNNs which are a classifier, a feature extractor, and an end-to-end learner. In this paper, we propose a novel method, Multi-channel PINN, to fully utilize sparse data in terms of representation learning. Although the number of publicly available CPI data has grown rapidly, public data is still sparse and has a large number of measurement errors. However, such techniques commonly require a considerable volume of dense data for each training target. Deep neural networks (DNNs), which have recently been applied to predict CPIs, performed better than other shallow classifiers. Machine learning’s advances in predicting CPIs have made significant contributions to drug discovery. In vitro experiments are commonly used in identifying CPIs, but it is not feasible to discover the molecular and proteomic space only through experimental approaches. Analysis of compound–protein interactions (CPIs) has become a crucial prerequisite for drug discovery and drug repositioning.
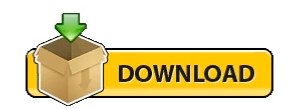